AI in QA Operations: Steps, Benefits, & Best Practices
In This blog
It’s nearly impossible to go through a workday these days without AI hearing people talking about AI and the changes it promises to bring to every area of our lives and across different industries. And guess what? It doesn’t skip the QA world. If you plan to attend a software testing conference in 2024, chances are AI will be stealing the spotlight. But why all the fuss?
Well, it’s simple. People and companies alike are eager to tap into AI’s potential to revolutionize how we do business. According to the 2024 State of Testing survey 60% of companies nowadays are still not using AI as part of their testing activities, but, we should expect this to change over the next couple of years as companies are constantly on the look of how to improve testing efficiency, and AI presents a compelling solution to complete testing tasks faster and more effectively than ever before.
In this article, we will explore the impact of AI on the QA world, the benefits it presents, and how to effectively implement and leverage it.
What is AI in QA Operations
AI in quality assurance represents the convergence of advanced technologies aimed at optimizing and enhancing software testing processes. At its core, AI leverages sophisticated algorithms and computational power to mimic human-like intelligence, enabling systems to learn from data, recognize patterns, and make decisions autonomously.
One key component of AI in QA is machine learning, a subset of AI that focuses on enabling systems to learn from data and improve their performance over time without being explicitly programmed. Machine learning algorithms can analyze historical data to identify patterns and trends associated with software defects and failures. Based on this, QA teams can use those insights to prioritize testing efforts on high-risk areas likely to encounter harmful issues, ensuring more effective resource allocation and risk mitigation strategies.
Other important AI aspects are Natural language processing (NLP) and Generative AI. NLP enables systems to understand and interpret human language, while Generative AI in quality assurance learns from existing data and focuses on creating new data. Both of those advanced AI components can contribute to improving testing processes by creating comprehensive, relevant test cases to ensure adequate test coverage for example.
AI in QA automation can also be a key component in enabling the execution of complex and time-consuming testing tasks with minimal human intervention. AI can boost the abilities of automation tools to the next level, allowing testing teams to generate scripts and execute test cases across different platforms and environments, significantly accelerating the testing process and reducing manual effort.
Steps to Implement AI in QA Operations
Here are the key steps to successfully implement AI in QA operations:
-
Assess Organizational Readiness: Start by evaluating your organization’s current QA tools, processes, and capabilities to determine readiness for AI integration. Identify areas where AI can contribute to your work and potential challenges, and understand who are the staff members who will regularly use it.
-
Define Areas for Improvement: Clearly define your objectives and areas before implementing AI in QA operations. Determine what specific QA tasks or processes you want to improve with AI. Whether it’s related to accelerating testing cycles, better testing data management, or optimizing test creation, establishing clear objectives will guide your AI implementation strategy and measure its success.
-
Select Appropriate Tools: Research and evaluate different tools and technologies that offer the capabilities and features required to address your organization’s specific QA needs effectively. Consider factors such as functionality, scalability, compatibility with existing systems, and ease of integration.
-
Invest in Staff Training: Ensure your QA staff members are equipped with the knowledge and expertise needed to leverage the chosen tools effectively.
-
Monitor and Optimization: After successfully implementing the new tools, continuously monitor and evaluate its performance to identify areas for improvement and optimization. Collect feedback from QA professionals and stakeholders to assess the effectiveness of AI tools and technologies in meeting business objectives and QA requirements.
Benefits of AI in QA Operations
Implementing AI in QA operations offers a myriad of benefits that contribute to more efficient, effective, and reliable software testing processes. Let’s take a closer look at some primary benefits:
-
Time-Saving: AI can streamline testing processes and enable QA teams to complete testing cycles faster. By automating time-consuming tests, AI reduces manual quality assurance efforts, saves valuable time, and allows QA professionals to focus on higher-value activities such as test design, exploratory sessions, and process optimization
-
Better Test Planning: AI-powered analytics enhance test planning by providing valuable insights into testing priorities, resource allocation, and risk assessment. By analyzing historical data, AI helps QA teams prioritize testing efforts effectively, focus on high-risk areas, and optimize test case execution strategies, ultimately improving the overall effectiveness and efficiency of test planning processes.
-
Enhanced Test Coverage: AI-driven testing techniques enable comprehensive test coverage by generating a diverse set of test cases that cover various scenarios and edge cases. By analyzing vast amounts of data and identifying potential risk areas, AI ensures thorough testing of software functionalities, reducing the likelihood of critical defects slipping through production and impacting end-users.
-
Scalability: AI tools are highly scalable and adaptable, capable of supporting organizations of any size to scale up when needed. Whether testing a small-scale application or a large enterprise system, AI-powered QA solutions can scale to meet the evolving needs and demands of the organization, ensuring consistent and reliable testing across diverse environments and platforms.
Challenges of Implementing AI in QA Operations
While the benefits of implementing AI in QA operations are substantial, organizations should pay attention to several challenges and considerations to ensure successful integration. Let’s explore some of the key factors to be mindful of:
-
Data Quality and Availability: Since AI algorithms rely heavily on data, QA teams need to ensure the quality, relevance, and availability of their data for AI tools to contribute to improved QA processes. In case AI tools get irrelevant data or only partially accurate data, it can harm its outcomes.
-
Integration with Existing Tools: As Organizations regularly use various tools, they may encounter compatibility issues when trying to integrate new AI tools. Even resistance to change from stakeholders can be a challenge here. Looking for tools that can integrate with other existing systems is essential, in addition to effective cross-department collaboration to ensure seamless integration into existing workflows.
-
Regulatory Compliance: Certain organizations operating in regulated industries such as healthcare, finance, and automotive must ensure compliance with relevant regulations. Those companies should verify before implementing a new AI tool that it complies with needed regulations and requirements.
Best Practices for Leveraging AI in QA Operations
These are four key best practices to guide you in leveraging AI for QA testing success:
Define Your Needs Clearly
Before integrating AI into QA operations, Identify the specific QA challenges you think AI can assist and that you want to implement as part of your workflow such as accelerating test case execution, improving testing data management, etc. Once objectives are defined, prioritize them based on their strategic importance and then start looking for tools if needed.
Look Beyond the Hype
Once you start actively searching for a tool, you will find a wide range of tools claiming it’s AI, or at least, include some AI features, it’s crucial not to be swayed by buzzwords. Instead, focus on understanding the actual value each tool offers. Evaluate and compare AI solutions based on factors such as functionality, scalability, and compatibility with existing workflows. Choose tools that align with your specific requirements and can deliver concrete benefits to your QA processes.
Monitor and Optimize Continuously
Implementing a new tool (whether it’s AI or not) is an ongoing process that requires continuous monitoring and optimization. Start by gathering feedback from QA professionals and stakeholders to understand whether the QA work is really improved thanks to the tool or it doesn’t bring any value. In addition, Depending on the tool you chose and its purposes, monitor key metrics such as testing velocity and defect escape rate to assess its effectiveness from a different angle. Use both ways to refine and optimize your QA processes in later testing cycles.
Keep up with AI Evolvement
Staying informed about the continuous evolution of AI is crucial. Attend industry conferences, engage with thought leaders, and explore professional events to stay updated on the latest advancements of AI in general and in testing techniques and tools in particular. Look for new opportunities and innovations that have the potential to improve your QA operations and stay ahead of the curve.
To Sum Up
In conclusion, the integration of AI into QA operations represents a significant opportunity for organizations to enhance the efficiency and effectiveness of their software testing processes. However, successful implementation requires planning and assessing your organization’s needs as well as consideration of challenges and considerations including integration with existing tools and regulatory compliance.
By embracing the best practices detailed in this article, organizations can harness the power of AI and leverage it for better, more effective testing processes. As AI continues to evolve, organizations must remain agile and adaptable, continuously exploring new opportunities and innovations to drive continuous improvement and look for new ways to deliver excellent software products to their customers.



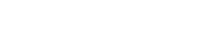
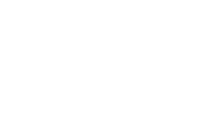
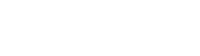


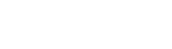
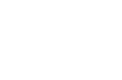



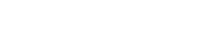
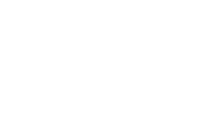
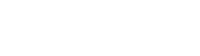


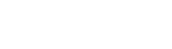
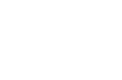